Last week I was fascinated to see how the media and many American CEOs reacted with horror to the news that a new and cheaper model would be just as good as OpenAI.
First of all: about 4 months ago, the Qwen team, which belongs to $BABA (+0,74 %) released the Qwen 2.5 model series. These ranged from 0.5b to 72b. The 72b model achieved far better performance than any existing open source LLMs on the market, including Meta Llama 3.3:
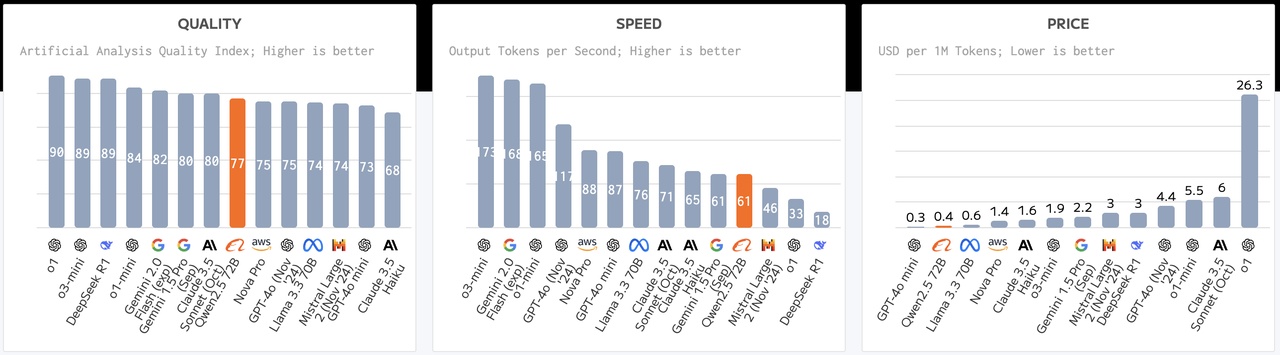
As you can see the performance is only conditionally worse, depending on which benchmark you take, it is even better than models from Anthropic's Claude, OpenAI's GPT-4o and Google's Gemini. How much of a stir has this caused: 0.
At least in the media. For us developers, however, the constant progress of open source models is a blessing, because in the case of qwen2.5:72b, for example, we download the models almost completely once and run them on private servers so that they work locally, cut off from any data. This means that no data can flow back to the provider OpenAI via an API request such as with OpenAI's models (actually a joke that they are called OpenAI) and you cannot use this data to retrain your models. This is essential, especially for applications with critical data.
By the way, if you would like to run your own personal assistant on your computer, you can do this with the open-source software Ollama, for example. If you don't know how much your laptop can handle if you don't have a graphics card with 64GB RAM installed, here is a brief overview:
- <=3b models approx. 5GB RAM (e.g. LLama 3.2 3b, qwen2.5:3b)
- 7/8/9b models approx. 16GB RAM (e.g. qwen2.5:7b, llama3.1:8b)
- <=70b models approx. 64GB RAM (but ultra slow on CPU only, then needs a Mac with Apple Silicon or something)
So to summarize: even with 3 year old Windows computers with 32GB RAM and an Intel or AMD processor you can run LLMs.
ollama link: https://ollama.com/
So back to the topic: why has nobody said anything about Qwen's success?
Maybe simply because Qwen's success had nothing to do with major tweaks in the model architecture. The fact is that the DeepSeek team made a few crucial changes to the model architecture, mainly 2 of them:
Instead of training the base model with supervised finetuning, i.e. in simple terms with labeled data set (good answer/bad answer), they trained it purely with reinforcement learning (mathematically programming in a kind of reward when desired training successes are achieved)
In the reinforcement learning approach, they also made crucial changes and established a new technique (Group Relative Policy Optimization GRPO), which accelerates the feedback process of positive learning in a very simplified way.
paper: https://arxiv.org/pdf/2501.12948
Now to the most important part:
Why DeepSeek "left out" a part and why it can be very good for us investors
In a detailed article, semianalysis has listed once again why certain numbers don't make as much sense.
TL;DR:
- $6M "training cost" is misleading - excludes infrastructure and operating costs ($1.3B server CapEx, $715M operating costs)
- Get ~50,000+ GPUs (H100/H800/H20) via detours (H100 forbidden GPUS!)
- They could also just offer inference cheaply, like Google Gemini, (50% cheaper than GPT-4o) to gain market share
- An export wormhole apparently enabled a $1.3bn expansion before H20 export restrictions
- DeepSeek R1 "reasoning" is good, but Google Gemini's Flash 2.0 is cheaper and just as good at least (from professional experience: the Gemini models are now very good)
- in the operational costs that have not been disclosed, the salaries for top talent are estimated to be up to $1.3M USD (per capita)
source: https://semianalysis.com/2025/01/31/deepseek-debates/
ASML CEO says so:
"In Fouquet's perspective, any reduction in cost is beneficial for ASML. Lower costs would allow artificial intelligence (AI) to be implemented in more applications. More applications, in turn, would necessitate more chips."
source: https://www.investing.com/news/stock-market-news/asml-ceo-optimistic-over-deepseek-93CH-3837637
Personally, I have a similar view that AI will become cheaper and better in the future, but chips will also have to be better and, above all, more chips will have to be produced. Without going into too much detail, this was a good opportunity, for example. $ASML (+0,93 %) , $TSM (+0,12 %) , $NVDA (-1,24 %) and co. to buy.